A guide for accelerating data governance with cataloging
- Chapter- 1 Introduction
- Chapter- 2 Data governance explained
- Chapter- 3 Need for robust data governance
- Chapter- 4 The data governance framework
- Chapter- 5 Governance with data catalog
- Chapter- 6 Data cataloging principles
- Chapter- 7 Best practices
- Chapter- 8 Benefits of an enterprise data catalog
- Chapter- 9 AI governance for successful ML initiatives
1. Introduction
The convergence of data, analytics, and artificial intelligence (AI) is revolutionizing industries, reshaping business strategies, and creating new growth opportunities. The surge in interest is further fueled by advancements in generative AI, with 40% of organizations planning to boost AI investments, according to McKinsey’s latest State of AI report1. While these trends signal a growing commitment to data-driven decision-making, many organizations continue to face challenges in unlocking the full value of their investments.
A key obstacle lies in the absence of a comprehensive and actionable governance framework for data and AI that spans applications from business intelligence to machine learning (ML). Gartner predicts that by 2027, 80% of organizations pursuing digital growth will struggle due to outdated governance practices2. The rapid evolution of generative AI and large language models (LLMs) further amplifies the urgency for modernized governance strategies that align with the complexities of today’s data landscape.
Effective data and AI governance are the foundational pillars of digital transformation. Organizations face numerous challenges, from managing diverse data sources and eliminating silos to ensuring data quality, secure access, and regulatory compliance. A forward-thinking governance strategy provides a clear pathway to navigate these complexities, enabling enterprises to maximize the value of their data and AI investments.
This guidebook underscores the importance of data governance for enterprises, strategies to achieve seamless and consolidated access to data assets, and how data catalogs act as a catalyst for simplified data management. As organizations adopt AI-driven data strategies, implementing a robust data governance mechanism is key to driving growth responsibly and sustainably.
2. Data governance explained
Data governance is a systematic approach that encompasses the principles, practices, and tools for managing an organization’s data throughout its lifecycle. By aligning data requirements with business objectives, it ensures better data management, quality, visibility, security, and compliance across the organization. An effective data governance strategy enables easy access to data for decision-making while safeguarding it from unauthorized access and ensuring compliance with regulations.
At the enterprise level, effective data governance involves clearly defining data ownership and control. It focuses on managing roles, responsibilities, and processes that ensure accountability and stewardship of organizational data assets.
3. Need for robust data governance
A well-defined data governance strategy is crucial for organizations to fully realize the potential of their data. By establishing strong data governance, businesses can effectively manage and utilize their data, ensuring it is accurate, secure, and compliant with privacy regulations. This not only helps companies gain a competitive advantage but also fosters customer trust by demonstrating a commitment to responsible data handling and privacy protection.
Other reasons include:
- Improved data reliability drives faster and more accurate decision-making across the organization.
- Elimination of data silos leads to uniform data usage and tighter collaboration across the organization
- Enhanced compliance with data regulations such as such as GDPR, CCPA and PII help in avoiding legal and financial risks
- Cost reduction through streamlined control mechanisms and efficient use of resources
- Increased operational efficiency by preventing data duplication and sprawl
- Improved security and privacy with measures to prevent unauthorized access to sensitive data
- Agility and scalability of business and IT with outlined processes for change
4. Data governance framework for enabling AI and analytics initiatives
A data governance framework is more like a blueprint that forms the basis for data strategy and compliance. It begins with a data model outlining data flows—such as inputs, outputs, and storage—and then adds rules, responsibilities, procedures, and processes to manage and control these data flows.
A well-managed data governance framework can successfully underpin an organization’s journey towards operating on digital platforms. However, creating such a framework requires a process to deal with common imperatives surrounding data, such as:
- Data scope: Identifying the categories of data (including master, transactional, operational, and analytical data), definitions or identification of data to be governed.
- Organizational Structure: Establishing clear roles and responsibilities, such as accountable owners, data leaders, IT teams, business units, and executive sponsors.
- Data standards and policies: Setting guidelines to specify what is the nature of data, location of the stored data and how it is used along with guidelines or standards for data ownership.
- Success metrics: Defining parameters to monitor the execution of the governance strategy and measure its success.
Building a robust data governance framework
Think of the model as a kind of blueprint of how data governance works in a particular organisation. And note that this governance framework will be unique to each organisation, reflecting the specifics of the data systems, organisational tasks and responsibilities, regulatory requirements, and industry protocols. An effective data governance framework must account for:
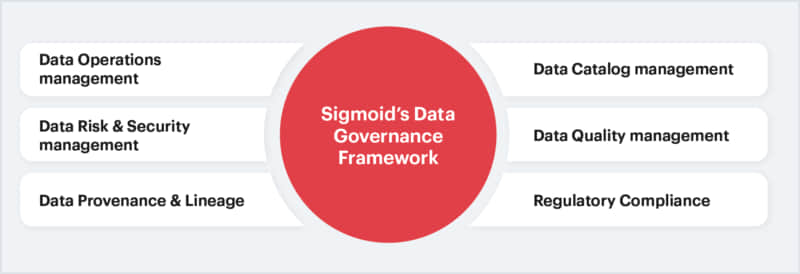
Fig 1: Sigmoid’s Approach to Data Governance
Data operations management
Data Operations Management ensures efficient data processing, storage, and movement while maintaining system reliability and performance. It streamlines workflows through automation and ensures consistent data availability. Key aspects include data provenance and lineage for tracking sources, updates, and errors across the data lifecycle, covering integration, extraction, transformation, replication, and virtualization to support business objectives.
Data risk management
Data risk management identifies, assesses, and mitigates potential risks to data integrity, security, and compliance. It involves proactive monitoring, incident response planning, and establishing controls to reduce vulnerabilities. Within a data governance framework, it safeguards organizational data against threats and ensures business continuity.
Data security management
Data security management ensures that data is protected from unauthorized access, breaches, and misuse. It involves implementing policies, controls, and technologies to safeguard sensitive information throughout its lifecycle. Key components include access controls, encryption, threat monitoring, and incident response protocols.
Data development
Data development focuses on the design, creation, and maintenance of data assets, ensuring alignment with organizational goals. It includes processes like database modeling, ETL workflows, and metadata management. As part of governance, it ensures structured, scalable, and usable data systems that meet evolving business needs.
Data catalog as means to achieve data discoverability
Effective data governance starts with understanding the organization’s data assets, and a data catalog plays a pivotal role in this process. By serving as a centralized repository of metadata, a data catalog enables stakeholders to quickly discover, understand, and access data. It provides a searchable index of available data, detailing its format, structure, location, and usage. Integrating a data catalog into governance enhances data management, fosters collaboration, minimizes redundancy, and ensures robust access controls and audit capabilities, streamlining data discovery, governance, and analytics activities.
Data catalog is driven by active metadata management. Metadata provides crucial context about data with information, such as:
- Data type
- Data classification
- Origins
- Current location
- Creation date
- Last updated on
- Change logs or revision history
- Owner and editors
Regulatory compliance
Regulatory compliance ensures data practices adhere to laws and standards such as GDPR, HIPAA, or CCPA. It involves documenting policies, conducting audits, and maintaining transparency in data usage. As part of the governance framework, it protects against legal risks while building trust with stakeholders.
Data quality management
Data quality management ensures data is accurate, complete, consistent, and reliable for decision-making. It includes processes to detect and resolve errors, monitor quality metrics, and maintain high standards. Integrated into data governance, it drives better insights and outcomes.
Data architecture management
Data architecture management focuses on designing and maintaining the structure and organization of data systems. It includes defining models, relationships, and integrations to support data flow and usability. As a governance component, it ensures scalability, efficiency, and alignment with business goals.
Data policy management
Data policy management establishes and enforces rules and guidelines for data usage, storage, and sharing. It defines responsibilities, access rights, and procedures to ensure consistent practices. Within governance, it drives compliance, standardization, and accountability across the organization.
5. Strengthening data governance with an enterprise data catalog
A data catalogue is a core component of data governance and an integral part of an organization's broader data analytics strategy. It makes use of metadata to provide organizations with a single, overarching view along with deeper visibility into their data assets. A data catalog is essentially a cluster of metadata combined with high-end data management and search tools. These search tools allow data users and analysts to locate specific data for intended use cases. This can help organizations efficiently manage their valuable data and get easy access to trusted data as and when required. Technologies like AI and machine learning have greatly diversified the use cases of metadata. Technical, business, and operational metadata have undergone a mini-revolution and have found usage beyond audit, lineage, and reporting.
A data catalog plays a significant role in supporting effective data governance efforts within an organization, by offering several key capabilities and benefits:
- Centralized metadata management: Stores detailed metadata, including lineage, quality, and ownership, aiding informed decisions about data usage, access, and compliance
- Comprehensive data inventory: Provides a centralized repository of all data assets, ensuring visibility into data location, ownership, and usage.
- Data classification: Classifies data by sensitivity and regulatory needs, enabling effective security measures and access controls.
- Data lineage tracking: Maps data flow from source to destination, ensuring accountability, quality, and compliance.
- Access controls & security: Integrates with governance policies to enforce secure, privacy-compliant access to data.
6. Data cataloging principles
These principles can be considered as basic guiding rules that must be followed by data catalog users. The following principles form the core of these set of directives for the users and catalogers:
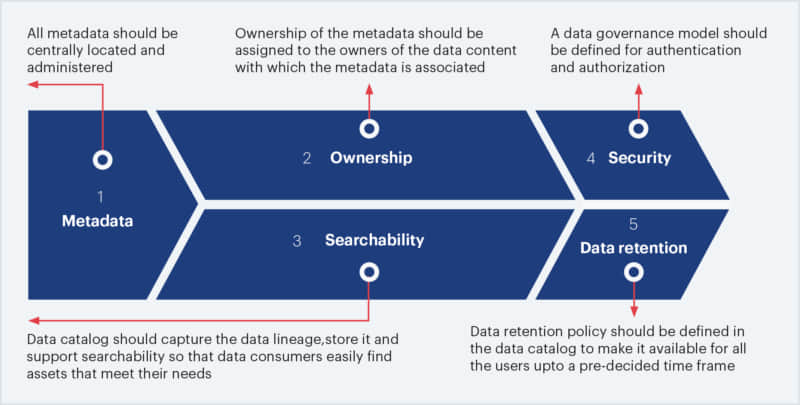
Fig 2: Data Cataloging Principles
7. Best practices to adopt data catalog
Intelligent data catalog adoption can ensure faster data discovery, less time to generate insights, and reduced time-to-market only if companies know the way to generate the greatest value from it. Here is a step-by-step process developed based on road-tested best practices to simplify the adoption of the data catalog for enterprises:
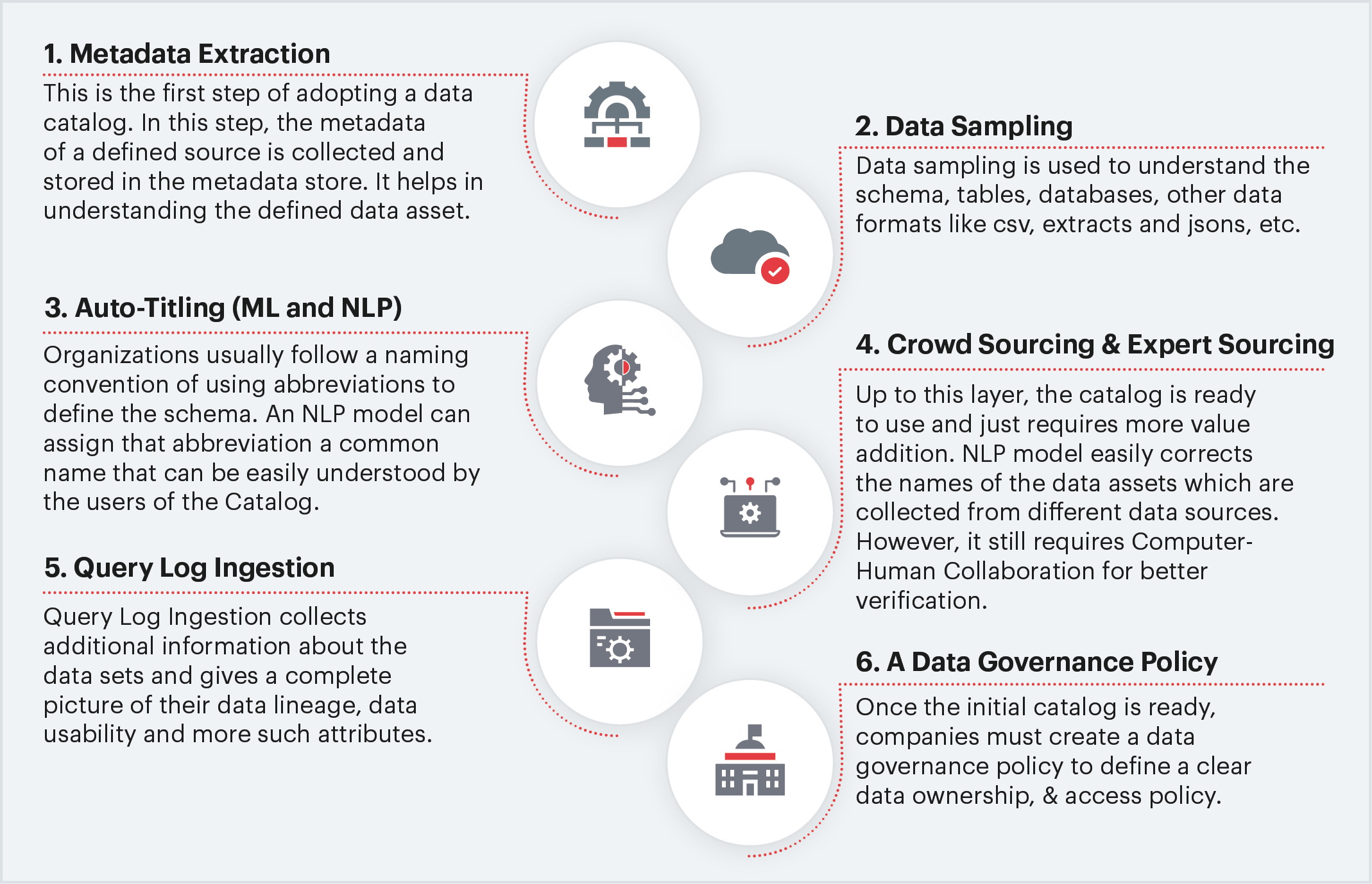
Fig 3: Best Practices to Adopt Data Catalog
8. Benefits of an enterprise data catalog
An enterprise data catalog reduces costs and saves time, enhances efficiency, streamlines governance, and accelerates decision-making, leading to improved innovation, seamless implementation, and fewer missed opportunities.
Advantage | How does it help |
---|---|
Flexible search |
A data catalog provides companies with flexible searching and filtering options. This allows data teams to find the required data sets using technical information, user-defined tags, or business terms in reduced time. |
Wider data access |
Data catalogs harvest multiple technical metadata from diverse connected sets of data. This facilitates deeper visibility and wider access across the enterprise. |
Business knowledge repository |
Metadata curation provides a way for data scientists to contribute business knowledge in the form of their business glossaries, descriptions, data profiles, classifications, tags, quality reports and more. |
Automated data processes |
Data catalog utilizes AI and machine learning to automate manual repetitive tasks. AI-backed metadata can augment cataloging capabilities with end-to-end data management. |
Improved governance |
Provides clear visibility into an organization’s data assets and their locations, ensuring easy tracking of data origins and storage practices for better availability, integrity, usability, and security. |
9. AI governance for successful ML initiatives
The core principles of governance—accountability, compliance, quality, and transparency—are just as essential for AI as they are for data. AI governance establishes a structured framework of policies and processes to guide the development, deployment, and management of machine learning (ML) models within an organization.
Proper governance ensures that AI systems align with ethical standards, regulatory requirements, and organizational goals while minimizing risks related to compliance, security, and reputation. Key aspects of AI governance include:
- Compliance and Ethics: Upholding ethical standards and adhering to legal and regulatory requirements for responsible AI use.
- Reproducibility: Ensuring ML models can be consistently reproduced for accuracy and reliability across environments.
- Explainability and Transparency: Providing clear insights into how AI models make decisions, enhancing trust and accountability.
- Model Deployment: Streamlining the deployment of models into production with robust serving capabilities.
- Model Monitoring: Continuously tracking performance to detect biases, drifts, or anomalies in real-time operations.
- Security: Safeguarding models against adversarial attacks and unauthorized access.
- Cataloging and Documentation: Maintaining detailed records of data, algorithms, and model workflows to support audits, updates, and collaboration.
With a well-designed governance framework, AI can drive business efficiency, optimize decision-making, and reduce operational risks while maintaining ethical and transparent practices.
Conclusion
Managing data in the age of data lakes and self-service can be quite challenging. However, today’s enterprises must ensure they have an effective data governance framework in place, aligned with their overarching data analytics strategy, to gain the most out of business data. To that, every organization must look to simplify data management and governance with a strong data catalog. It helps data scientists to get more value from their enterprise data assets and empowers them to leverage data in the way they had always wanted.
By adopting a modern data catalog business are essentially taking their first step to creating self-service analytics ecosystems to democratize data, implement data governance, accelerate digital transformation, and reduce time to actionable insight.
References
1. McKinsey, State of AI Report
https://www.mckinsey.com/capabilities/quantumblack/our-insights/the-state-of-ai-in-2023-generative-ais-breakout-year#/