Roadmap for Demand Planning and Forecasting
Empowering proactive decisions with data-driven demand planning- Chapter- 1Understanding demand planning and forecasting
- Chapter- 2Key steps for an effective demand planning process and forecasting
- Chapter- 3Best practices for demand planning and forecasting
- Chapter- 4Demand Planning and Forecasting Trends
- Chapter- 5Conclusion
- Chapter- 6Demand forecasting customer success stories
- Chapter- 7FAQs
With access to vast datasets (from sales, inventory, production etc.) and advanced analytics, businesses can now leverage demand forecasting to make more precise, proactive decisions, driving efficiency and staying ahead in a competitive market. It enables businesses to anticipate market changes, enhance customer satisfaction, and maintain a competitive edge by being proactive rather than reactive.
Amid economic uncertainties, efficient resource allocation and maximizing returns are more critical than ever. Demand forecasting serves as a reliable guide, helping businesses navigate an evolving global landscape with confidence.
Understanding demand planning and forecasting
Demand planning leverages historical data, patterns, and analytics to predict future demand for specific products within a set period. It enhances revenue forecast accuracy, aligns inventory with anticipated demand, and maximizes product profitability. Demand planning also guides product roadmaps, inventory production, and aligns stock levels to demand fluctuations.
Demand forecasting is a key component of end-to-end planning process. It enables leaders to anticipate market shifts, make proactive decisions, and stay responsive to evolving customer needs.
Why is demand planning and forecasting so critical?
Demand planning holds significance in business as an integral component of building a robust supply chain. It serves dual purposes—enabling companies to monitor inventory, ensuring the appropriate quantity of products is available at the right locations to meet customer demand, and facilitating more efficient inventory management.
An effective demand planning process offers benefits in various areas:
- Dynamic scenario planning: Adjusts sales and financial scenarios in real-time using leading indicators, enabling strategic modifications to portfolio cost structures.
- Resource allocation: Allows for tailoring offerings and investments in marketing, pricing, sales deployment, customer risk management, and cash collection in response to demand shifts.
- Operational Decisions: Optimize supply and production planning, production capacity, and inventory management.
During periods of high volatility, businesses must evaluate rapidly changing market forces and consumer demand to make informed decisions regarding commercial and customer investments. These decisions should be optimized across diverse product categories, industries, locations, and channels.
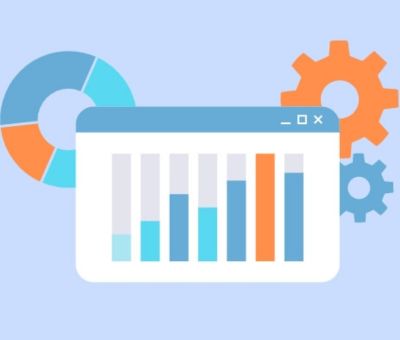
Demand forecasting for CPG
The ever-changing consumer behaviour coupled with increasing market volatility amidst the pandemic has forced organizations to build a resilient supply-chain model. Sigmoid’s demand forecasting services improve the efficiency of supply chain operations with inventory planning by identifying shifts in consumer behavior to enable SKU-level optimization for e-commerce.
Reduce your inventory costKey steps for an effective demand planning process and forecasting
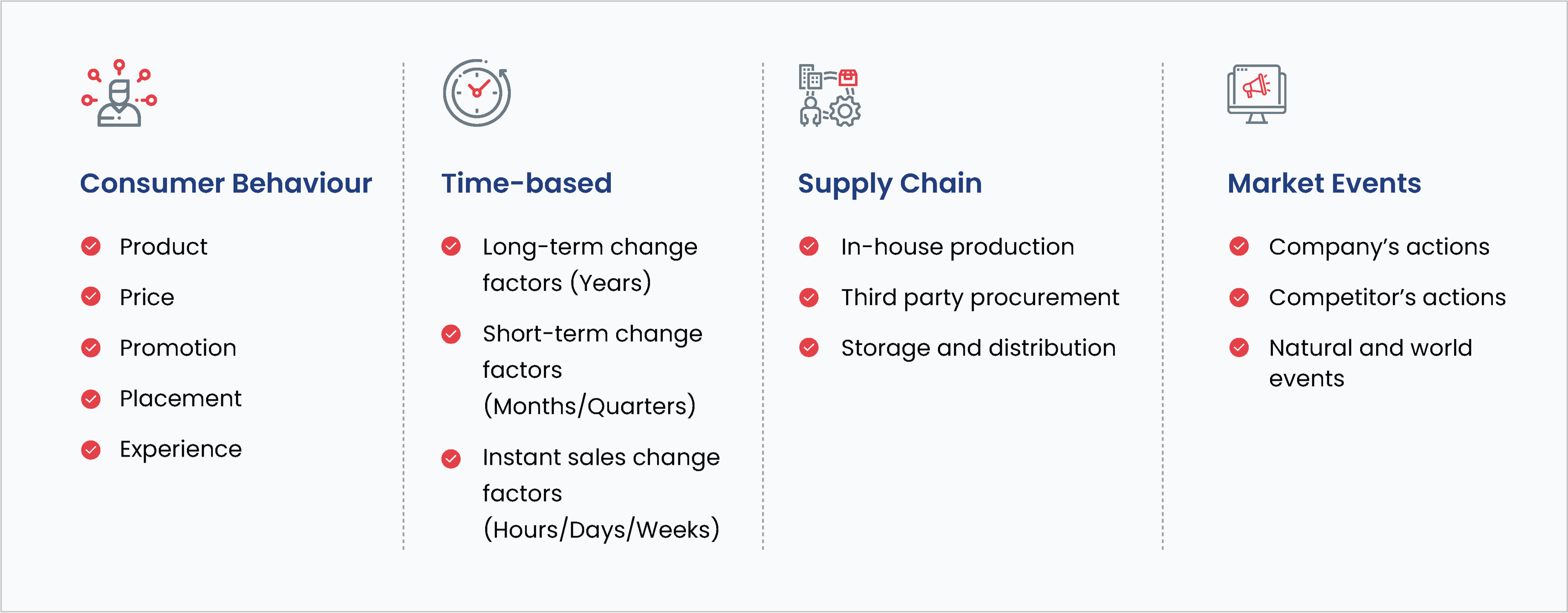
Fig.1. Factors influencing demand planning process
- Preparation of data: Collect data internally from sales, marketing and operational departments, including finance, production and procurement. Acquire historical data like sales forecast, historical sales data, point-of-sale (POS) terminal data, survey data, customer orders and other key metrics. Ensure that the data is complete, and representative of different market conditions. Accounting for internal information, like product attributes, inventory data, and safety stocks must be tied to real-time master data management to ensure that data is current and accurate.
- Initial forecasting: Apply chosen forecasting methods (qualitative or quantitative) such as time series analysis or machine learning algorithms to create an initial projection of future demand based on historical data by identifying patterns and trends. Incorporate qualitative insights, expert opinions, and market knowledge to supplement quantitative forecasts. Bring in external data like weather, economic factors, and consumer purchase behaviour to drive model results.
- Incorporation of market intelligence: Gather external market intelligence, including industry trends, competitor activities, and macroeconomic events. Adjust initial forecasts based on qualitative insights gathered from market research.
- Consider sales goals and financial reports: Integrate the forecasting process with business planning efforts and make forecasting a recurring process. Combine the initial forecast with insights from sales teams and regional managers who have a closer understanding of local market conditions. This helps reconcile the initial forecast with on-the-ground realities. Align the forecast with overarching financial and sales goals set by the leadership. This ensures that the demand plan is in line with broader organizational objectives.
- Refine a final forecast: Conduct collaborative demand planning meetings involving representatives from sales, marketing, finance, and other relevant departments for better perspectives, context and coverage. Discuss and refine the forecast based on the input from various stakeholders. Adjust the forecast iteratively, taking into account feedback, market changes, and any new information that becomes available.
- Creating a demand plan: Develop actionable strategies to align inventory levels, resources, procurement timelines, and production schedules with the forecasted demand. This step includes determining product quantities, setting inventory levels, and allocating stock to specific locations while integrating market intelligence and analyzing different scenarios to adapt to demand fluctuations.
- Performance monitoring based on real-time analytics: Use real-time data sources, such as point-of-sale data, to gauge current sales and demand trends. Define and track KPIs to measure the accuracy and performance of the forecast. Monitor performance in real-time using analytics tools to identify deviations and trigger timely adjustments.
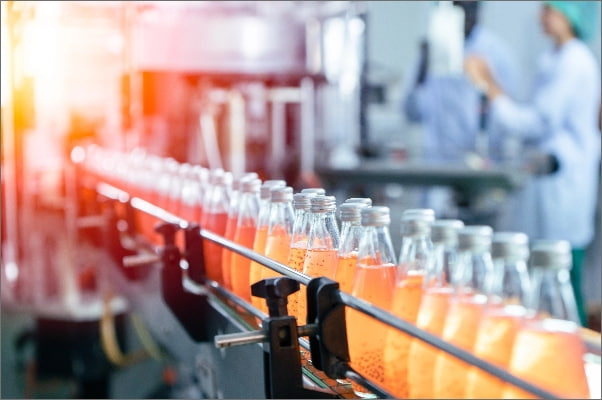
Demand forecasting solution delivers prediction accuracy of 92%
Sigmoid provided a customized demand forecasting solution utilizing advanced ML algorithms, incorporating market indicators and macroeconomic parameters. The solution led to 80% faster time to insights, reduced man-hours in demand calculations, and enabled swift responses to changing market dynamics.
Gain market insights to expand salesBest practices for demand planning and forecasting
Implementing the best practices including data science for demand planning can enhance overall process effectiveness and yield more precise forecasting outcomes.
- Carefully assemble data using advanced data mining and aggregation techniques
- Get stakeholder buy-in for incorporating statistical modeling into the process
- Use collaborative tools to integrate the forecast data
- Leverage AI or ML to generate a baseline forecast and fine-tune the models as per requirements
- Define and design process flows to ensure seamless execution
- Establish continuous monitoring practices to reduce errors and biases
AI-based Demand Forecasting in Action
Demand Planning and Forecasting Trends
- AI-Driven forecasting takes center stage
AI is revolutionizing demand forecasting by automating processes, leveraging unique datasets, and continuously learning to adapt to market changes. Businesses must integrate AI strategically to improve efficiency, accuracy, and responsiveness. - Privacy of unique data sources are crucial
With stricter privacy regulations, businesses are turning to publicly available and unique datasets that exclude personal information. Leveraging external data, like event intelligence or location-based insights, can offer a competitive edge in demand accuracy. - Demand variations across markets will grow
Emerging markets and multi-channel strategies are creating diverse demand patterns. Businesses must adopt localized forecasting approaches tailored to specific markets to optimize resource allocation and enhance profitability. - Real-time data integration becomes essential
Accessing dynamic, real-time data is critical for adjusting forecasts and responding swiftly to market shifts. Integrating APIs and real-time external data ensures organizations remain agile and competitive in a rapidly evolving landscape.
Conclusion
As AI/ML algorithms continue to evolve and advanced technologies emerge such as Gen AI, they can aggregate more data and identify the factors influencing and shaping demand. This makes it easier to adjust or enrich forecasts in real time, allowing for leaner inventory without missing the mark on demand. Consequently, companies can make better decisions, creating resilient supply chains that navigate disruptions and seize market growth opportunities.
A dedicated Demand Planning team, consisting of data scientists, analysts, and planners, can become a key asset for organizations seeking a strong competitive edge. For supply chain professionals, understanding how to implement artificial intelligence and machine learning programs can help them deploy a lean, agile, and data-driven approach to reveal new ways to cut costs and improve revenue.
Demand forecasting customer success stories
FAQs
Demand planning has much in common with demand forecasting, though most experts consider demand planning to go beyond statistical forecasting to include planning the scope of the inventory. Both terms are sometimes used as synonyms for demand management, but they are usually regarded as distinct components of it. Demand management also includes demand shaping, the process of trying to affect demand through price changes, product substitution, promotions and other methods.
Demand planning is often conducted in the early stages of sales and operations planning (S&OP), a process for aligning supply with demand by coordinating sales planning and forecasting with production planning in a unified approach. Timely planning enables companies to make sure supply meets any expected demand fluctuations. It also informs the manufacturing process, while ensuring the capacity and availability of materials to meet projected production goals.
- Product Portfolio Management: Product portfolio management oversees the overall product lifecycle, beginning with the introduction of a new product through to its end-of-life planning. In many cases, product lines are interdependent, and understanding how new products may influence demand for other products is important to understand the overall product mix required to maximize market share.
- Statistical Forecasting: Using historical data, statistical forecasting creates supply chain forecasts with advanced statistical algorithms. In this area, it is important to determine the accuracy of each model, identify outliers and exclusions and understand assumptions.
- Trade Promotion Management:Trade promotion or marketing events use special events (such as discount prices or in-store giveaways) to spike consumer demand. These activities can influence demand, especially in the retail industry.
- Demand sensing: Demand sensing uses a combination of new sources of data, such as weather, infectious disease trends, government data and more, with historical trend data and applies AI to detect disruptions and demand influences in near real-time.
- Improving accuracy and time-to-market
- Increased customer satisfaction
- Agile supply chains
- Improved resource allocation and investments based on shifts in demand
- Better operational decisions
- When the marketing data is excluded, the error margin of demand predictions may increase since marketing data and marketing effectiveness have a big impact on future sales.
- New products don’t have sufficient historical data. That’s why accuracy expectations from the forecast models should not be high for newly released products.
- If the supply chain network is not connected, the business will ultimately struggle to obtain the true value of demand forecasting. The purpose of demand forecasting is to make changes to optimize the entire supply chain.
- Forecasts can only be as good as the input data. Companies need to ensure that important changes are reflected in real-time in their data sources.