The top Data Engineering trends to look for in 2025
Reading Time: 4 minutes
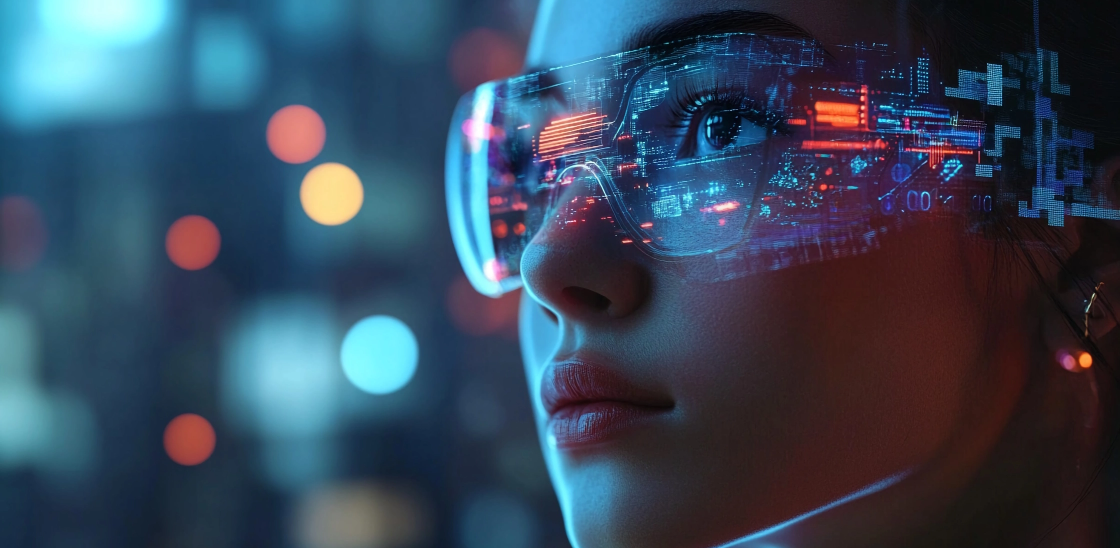
The global big data and data engineering services market size is predicted to be worth $276.37 Billion by 2032, fueled by rapid advancements in AI, cloud computing, and decentralized architectures¹. These technologies are helping organizations innovate, scale, and drive unparalleled growth by transforming how they manage and utilize data. In this blog, we’ll explore four key data engineering trends poised to shape the data and analytics landscape in 2025, driving innovation and competitive advantage.
Trend 1: Data lakes and meshes work together to democratize enterprise data
The synergy between data lakes and data meshes is reshaping enterprise data strategies. While data lakes provide scalable, cost-effective storage for raw and semi-structured data, data meshes decentralize data ownership, aligning it with business domains. Together, they form a complementary ecosystem where lakes handle ingestion and storage, while meshes enable agile, domain-specific access and governance.
Data mesh is a paradigm shift in analytical data management that addresses some of the limitations of data warehousing and data lakes. It is founded on the principles of treating data as a product—designing it to be easy to use, tailored to users, and operationalized. It enables a self-serve data platform to provide employees seamless access without depending on a central team. By adhering to federated computational governance companies can establish unified rules and standards for privacy, access controls, quality, and compliance. Together, data lakes and meshes form a complementary ecosystem where lakes provide scalable storage, and meshes enable decentralized, domain-focused data access, creating a balanced and innovative approach to enterprise data management.
Trend 2: Generative AI accelerates platform modernization
Generative AI streamlines legacy system modernization by automating migration and transformation workflows. It can identify redundant code, map dependencies, and recommend optimizations to transform legacy environments. Generative AI further enables automated ingestion and transformation processes, ensuring seamless transformation of legacy systems into modern architectures. This might involve converting legacy code into cloud-compatible formats, streamlining data pipelines, and using automation to validate transformed datasets.
Through these automated processes, businesses can migrate data and workloads more efficiently while reducing technical debt and accelerating innovation. By leveraging features such as automated ETL (extract, transform, load) processes or real-time testing, enterprises can build robust and scalable platforms. These platforms are designed to thrive in data-driven ecosystems, ensuring agility and minimal risks throughout the modernization journey.
Trend 3: Automation of data governance with AI
AI-driven automation transforms data governance by streamlining processes such as policy enforcement, metadata management, and access control. Generative AI-powered tools ensure clean and reliable datasets through intelligent monitoring, anomaly detection, and real-time corrections, enabling faster and more accurate insights.
Additionally, AI-powered tools provide comprehensive visibility into data lineage, tracking its journey from origin to consumption and supporting regulatory compliance. These systems can document past transformations to optimize future data flows, enabling sustainable growth. By integrating AI into data pipelines, businesses can unlock faster insights, improve accuracy, and build operational resilience, paving the way for a more efficient and data-driven future.
Master data management (MDM) is also undergoing a transformation with AI-powered automation. Innovations like automated label extraction and data consolidation ensure consistency, simplify compliance with regulations, and enhance decision-making. These advancements make it possible to manage complex datasets more effectively, unlocking efficiencies in areas such as Product Information Management (PIM) and regulatory adherence.
Trend 4: Expansion of IT across business operations
The integration of IT into factory floors is unlocking real-time insights and predictive analytics to enhance operational efficiency. For instance, IoT sensors integrated with AI platforms provide granular monitoring of machinery, enabling predictive maintenance to reduce downtime by identifying faults before they occur.
In customer interactions, AI-driven sentiment analysis tools analyze real-time customer feedback from emails, chats, and social media to deliver hyper-personalized responses. Generative AI enhances these tools by crafting context-aware messaging, ensuring quicker and more relevant customer support.
In data interfaces, generative AI streamlines data consolidation across disparate systems, ensuring clean, consistent, and accurate data for decision-making. Automated label extraction from complex datasets speeds up regulatory compliance processes, while AI-driven Product Information Management (PIM) tools simplify the categorization and retrieval of product data.
Conclusion
The future of data engineering is not just about technological advancement, but about strategic transformation. Generative AI has rapidly evolved from a novel technology to a critical operational enabler, fundamentally redesigning how organizations approach data infrastructure. As businesses navigate increasingly complex data landscapes, the integration of AI-powered solutions will become less of an option and more of a necessity.
From automating legacy code migration to enabling real-time data observability, generative AI is creating a new paradigm where data becomes more intelligent, accessible, and actionable. Enterprises that can effectively harness these AI-driven capabilities will seamlessly optimize their current data ecosystems and position themselves at the forefront of innovation, turning data from a technical resource into a strategic competitive advantage.
Reference
1. https://www.marketdataforecast.com/market-reports/big-data-engineering-services-market
Featured blogs
Talk to our experts
Get the best ROI with Sigmoid’s services in data engineering and AI