Overcoming data challenges for accurate demand forecasting in MedTech
Reading Time: 5 minutes
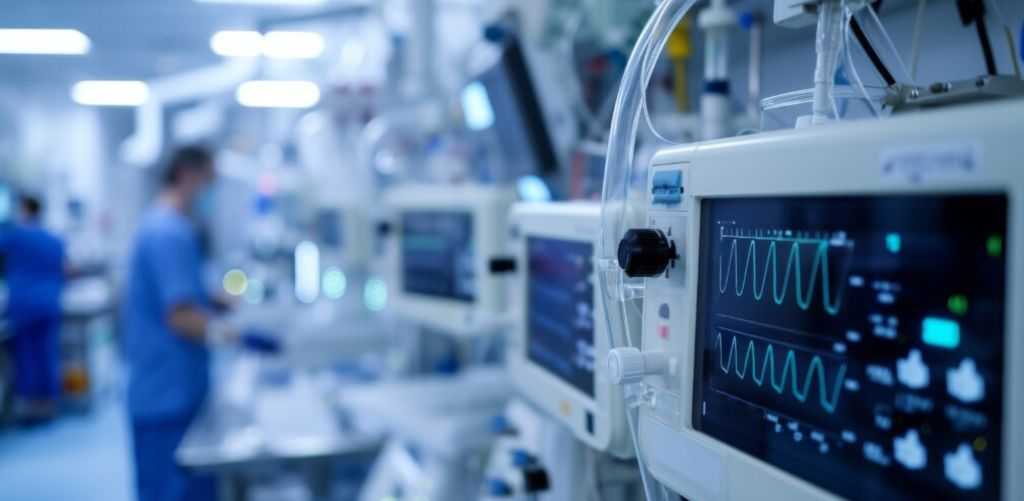
Introduction
In a high-stakes industry like MedTech, mastering accurate AI- driven demand planning and forecasting is critical. If planners fail to accurately match supply with demand, the results are product shortages, scrapped inventory, cancelled procedures and patient health risks.
However, demand forecasting in MedTech is quite a complex process due to a number of factors such as vast product portfolio, pricing and contract terms variability, and reliance on fragmented data. Demand forecasting is also impacted by a lack of upstream (manufacturers, suppliers) and downstream (hospitals, care facilities) channel visibility, inability to account for signals that influence demand such as shelf life, product serialization, new product introductions, changing regulations, supply chain disruptions, public health emergencies, consumer demographics etc. and outdated IT systems that fail to provide a complete picture.
In this blog, we’ll examine the challenges to MedTech forecasting and outline strategies to address them for higher accuracy.
Understanding the Difficulties of Demand Forecasting in MedTech
- SKU-level complexity: MedTech companies handle vast product portfolios that include capital equipment, such as MRI machines, alongside consumables used with these devices. Each SKU has unique demand patterns and dependencies that interact in ways traditional forecasting cannot easily capture. For example, an increase in MRI machine sales typically boosts demand for related consumables. A lack of detailed SKU-level forecasting often leads to misaligned inventory and production, disrupting supply chains and affecting revenue.
- Pricing and contract terms variability: MedTech companies serve diverse customers, from hospitals to integrated delivery networks (IDNs), healthcare organizations (HCOs) and ambulatory surgical centres (ASCs). Each client may have customized contracts with specific volume commitments, discounts, pricing structures and payment terms. Accurately translating units sold into revenue requires deep insights into contract details, which are often scattered across different systems. These variabilities are difficult to factor into forecasts, which can lead to discrepancies in revenue projections.
- Complexity in capital equipment forecasting: Capital equipment and consumables are highly interdependent, so it’s critical to forecast capital equipment pipelines. For example, a rise in MRI machine sales would typically lead to an increase in demand for the consumables used in MRI procedures. Forecasting demand for such capital equipment and its associated consumables accurately is critical, as errors in capital equipment sales forecasts can ripple through the supply chain. The high price points and intermittent capital equipment sales make forecasting complicated.
- Regulatory and compliance complexity: Regulatory bodies such as the FDA (United States), EMA (European Union), and other regional authorities enforce distinct compliance standards, timelines, and approval processes. Additionally, emerging markets often have their own evolving regulatory environments, further complicating global forecasting efforts. The compliance journey often involves lengthy approval cycles, extensive clinical trials, and rigorous documentation. These delays can shift product launch timelines, making it difficult to align manufacturing schedules, inventory planning, and distribution strategies with actual market demand.
Data issues that impede demand forecasting in MedTech
Fragmentation of data | Data across departments are often fragmented and stored in isolated systems, preventing a comprehensive view of demand factors. This restricts a holistic demand forecast considering all relevant customer and business factors. |
---|---|
Dependence on historical data | Historical sales data is commonly used as the primary input for forecasting, but without integrating data on pricing, contracts, market demand, and customer needs. This data alone may not give a complete picture as it overlooks critical variables. |
Limited collaboration | Data silos prevent teams from sharing insights and information with other internal or external stakeholders, creating many blind spots. Gaps in data flow prevent the alignment of production and inventory with real-world demand. |
Restricted data due to regulations | Limited availability of critical data, such as patient outcomes or real-world product performance due to stringent regulations. These regulatory constraints delay or restrict access to valuable information needed for accurate forecasting. |
Data structure complexity | Analysis and integration of both structured data, such as sales figures and inventory levels, and unstructured data, like clinical trial results, physician notes, or regulatory documentation can be challenging, often resulting in missed insights. |
How to improve demand forecasting accuracy for MedTech businesses
To allow for continuous innovation and scalability, legacy infrastructures need to be modernized. Such digital transformations can be led by data and technology. Some of these initiatives may include:
Data modernization and management
Leveraging advanced analytics and AI
AI-powered predictive analytics solutions helps MedTech companies analyze complex datasets, uncovering patterns that traditional methods might miss. Machine learning algorithms can generate forecasts that dynamically adjust based on emerging trends, such as fluctuating market demands or supply chain disruptions. For example, predictive models can analyze past sales, economic indicators, and contract data to provide SKU-level forecasts that align more closely with actual demand.
Using GenAI to automate contract analysis
With pricing and contract terms as complex as they are in MedTech, AI tools such as GenAI models can redefine the future of forecasting process. GenAI can automate the extraction of key information from contracts stored in various formats, such as PDFs or scanned documents. This process allows for faster and more accurate conversion of units sold into revenue, factoring in each contract’s specific terms. Automated contract analysis not only saves time but also reduces the risk of human error in handling contracts, enhancing the precision of revenue forecasts.
Establishing collaborative and integrated data-sharing
Implementing cloud-based dashboards and real-time data-sharing tools ensures that teams can access the same up-to-date information. By establishing collaborative forecasting processes, MedTech companies can enhance communication between sales, R&D, and supply chain teams, allowing each department to share critical insights that inform more accurate forecasts. Improved data-sharing also enhances transparency, especially with key supply chain partners like tier-1 suppliers and customers. By aligning internal and external data streams, MedTech companies can better anticipate changes in demand, respond to supply disruptions in real time and improve overall forecast accuracy.
Conclusion
The MedTech industry is witnessing rapid data growth with the rise of connected devices and smart systems. This data surge presents both a challenge and an opportunity—companies that effectively manage and harness this information will be better positioned to create accurate demand forecasts, optimize supply chains, and maintain a competitive edge. Data modernization along with the use of advanced technology can transform how MedTech companies approach demand planning. At Sigmoid, we empower MedTech companies to implement unified data systems and design sophisticated forecasting models for making smarter, data-driven decisions across all facets of their operations.
Featured blogs
Talk to our experts
Get the best ROI with Sigmoid’s services in data engineering and AI