Enhancing liquidity risk control with next-gen data management solutions
Reading Time: 7 minutes
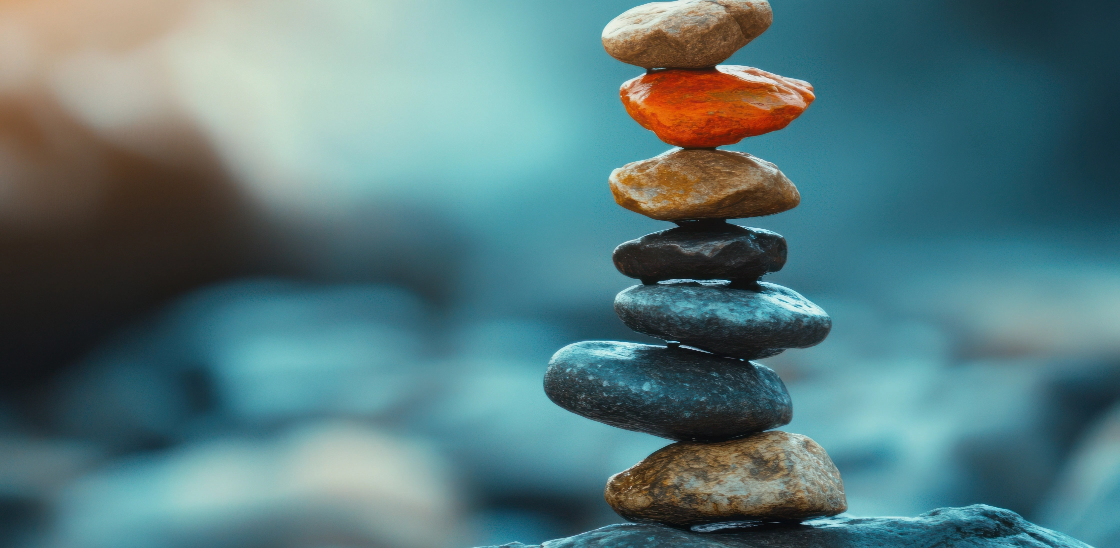
Banks need data-driven frameworks that enable real-time insights, precise stress-testing, and proactive risk mitigation to ensure operational resilience, regulatory compliance, and robust liquidity management. According to a recent survey, 96% boards or chief financial officers view enhancing liquidity risk management as a critical mandate. However, many institutions struggle with a lack of deep domain expertise, hindering their ability to interpret and manage these complex risks effectively.
High-Quality Liquid Assets (HQLA) play a crucial role in classifying and safeguarding liquidity, especially during times of market stress, economic crises, or regulatory scrutiny. For banks, it is essential to have a robust strategy in place to ensure they meet liquidity requirements and maintain solvency under pressure. However, without data preparedness and technical agility, institutions may find it challenging to classify, track, and leverage these assets to optimally drive key decision-making insights.
Understanding HQLA and its importance
HQLA (High-Quality Liquid Assets) refers to assets that can be easily and quickly converted to cash with minimal loss in value, especially during times of financial stress. HQLA is a core component in calculating Liquidity Coverage Ratio (LCR), which regulatory frameworks, such as Basel III, require banks to maintain. These assets are essential to ensuring that financial institutions have enough liquidity to cover their short-term obligations in times of market volatility.
What Qualifies as HQLA?
HQLA are categorized into three tiers based on their liquidity and risk profile:
- Level 1 Assets: These are the most liquid and least risky, including cash, central bank reserves, and sovereign bonds from highly rated countries. They are not subject to any haircut or cap, meaning banks can fully count them toward their liquidity requirements.
- Level 2A Assets: These include highly rated corporate debt securities and covered bonds, which must meet specific liquidity standards. However, these assets are subject to a haircut—typically 15%—to account for potential value fluctuations under stress.
- Level 2B Assets: This category includes lower-rated corporate bonds and certain equities. These assets are riskier and less liquid, so they face a higher haircut (25% to 50%) and can only compose a small portion of a bank’s total HQLA portfolio.
Regulatory requirements: Basel III and the Liquidity Coverage Ratio (LCR)
The Basel III framework, developed in response to the 2008 financial crisis, introduced the Liquidity Coverage Ratio (LCR) to ensure that banks maintain an adequate stock of HQLA. The LCR requires banks to hold enough HQLA to cover their total net cash outflows over a 30-day stress period, thereby providing a buffer against short-term liquidity disruptions. The formula is as follows:

By mandating that banks maintain a sufficient stock of liquid assets, Basel III ensures that institutions are better equipped to meet their financial obligations during periods of market turmoil. Failure to comply with LCR standards can result in regulatory penalties, and in extreme cases, could threaten a bank’s solvency.
The significance of HQLA goes beyond regulatory compliance. By maintaining a robust HQLA portfolio, banks can weather unexpected liquidity demands without being forced to sell off illiquid or distressed assets at fire-sale prices, a scenario that could exacerbate a financial crisis. As liquidity risk continues to dominate regulatory agendas, HQLA plays a critical role in fortifying the resilience of the global banking system.
Adapting to the 5G-6G HQLA classification changes
The liquidity classification framework for High-Quality Liquid Assets (HQLA) has seen significant updates in recent years, notably through changes to the Federal Reserve’s FR2052a reporting requirements. These updates aim to enhance the granularity and precision of asset classification, ensuring that banks are better equipped to meet their liquidity obligations and maintain stability during periods of financial stress.
Updates in HQLA classification: FR2052a and 6G Standards
In 2021, the Federal Reserve introduced an important revision to the FR2052a classification table, which is a critical component of liquidity risk management reporting. The FR2052a report tracks the inflows and outflows of cash, and its updates reflect a more nuanced approach to asset classification, particularly for HQLA. This was followed by the announcement of the 6G requirements, which introduce even stricter guidelines.
The new requirements compel banks to maintain a more detailed and dynamic approach to their asset classification and liquidity management strategies. The updated framework introduces new asset categories, refined classifications, and adds new items, demanding more precise data management and compliance efforts.
Key Changes in Asset Categories:
The proposed FR2052a Asset Category now includes 49 items, with 12 new items added. Some of the most important changes include:
- Equities:
- Fixed Incomes:
- New categories have been created for securities issued by central banks, helping to identify high-quality liquid assets more effectively.
- PSE (Public Sector Entities) securities, particularly non-U.S. securities, have their own classifications, reflecting the increasing globalization of asset portfolios.
- Municipal Securities (Munis):
- Mortgages:
A more granular breakdown of equity holdings has been introduced, distinguishing between common equities and fund shares. This additional clarity helps regulators and banks assess liquidity more accurately.
A new classification for non-investment grade municipal bonds has been introduced, distinguishing Revenue Obligation municipal securities from other asset classes. This provides greater insight into the risk and liquidity profile of these assets.
A new classification for mortgage-backed securities (MBS) has been introduced, distinguishing between agency and non-agency MBS. This allows for a clearer understanding of the risk exposure related to mortgage assets, enhancing liquidity and risk management capabilities.
The introduction of new FR2052a and 6G requirements demands a more proactive approach to compliance. Banks must invest in advanced data management to ensure accurate, real-time asset classification and reporting. The heightened granularity in HQLA mandates more detailed reports, while automating asset classification reduces the risk of errors, helping banks meet regulatory standards and safeguard liquidity in a shifting financial landscape.
The role of data modernization in HQLA management
Banks need data-driven solutions where data flows seamlessly from various sources—market prices, economic indicators, and internal assessments—into a unified platform that offers real-time insights into liquidity positions. This is the promise of data modernization. It empowers banks to make informed decisions, respond swiftly to market changes, and ensure that their asset classifications are accurate and aligned with evolving regulatory demands.
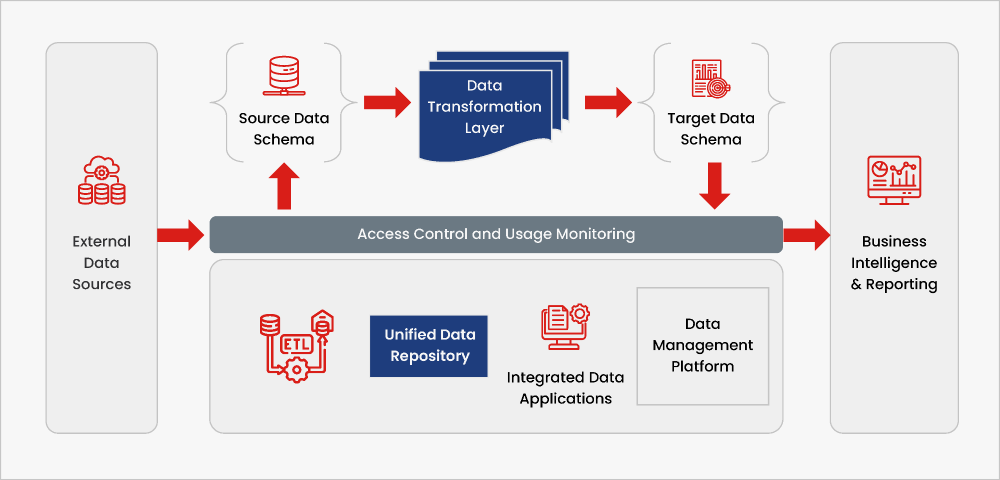
Fig 1. Data modernization workflow
- Real-time data collection and ETL process
- Data ingestion: Modernization enables banks to collect data from multiple sources—market prices, economic indicators, and internal assessments—through real-time ingestion pipelines.
- ETL Pipelines: Using powerful ETL (Extract, Transform, Load) processes, raw data is efficiently transformed and loaded, preparing it for further analysis.
- Rule definition and automated classification: Rules are set up to automate asset classifications based on liquidity characteristics, helping banks stay compliant with Basel III and other regulations.
- Centralized data storage and quality assurance
- Cloud Architecture: Cloud-based data lakes and warehouses support scalable and accessible data storage, enabling seamless collaboration across departments.
- Data Quality Checks: Automated quality checks ensure data accuracy by detecting inconsistencies or missing values, essential for accurate asset classification.
- User Acceptance Testing (UAT): UAT processes are built in to validate data quality and transformations before classification, minimizing errors and ensuring reliable data insights.
- Data transformation for HQLA classification
- Cleaning and Enrichment: Raw data is standardized and enriched to resolve issues like inconsistent identifiers, supporting accurate HQLA classification.
- Automated and Manual Classification: Data is classified based on liquidity—Level 1 assets (e.g., cash or government securities) or Level 2A/2B assets (e.g., corporate bonds)—through automation, with manual overrides for flexibility.
- Regulatory Compliance: Accurate classification enables banks to produce reliable HQLA reports, meeting the Liquidity Coverage Ratio (LCR) and other regulatory requirements.
- Automated risk reporting and performance monitoring
- Automated reporting: Automated reports deliver real-time insights into liquidity positions, minimizing manual data handling and reducing errors.
- Performance monitoring: Continuous monitoring ensures that the HQLA management process runs smoothly, with alerts for any issues in data quality or processing.
- Support mechanism: A support system addresses data inconsistencies or system issues promptly, ensuring that reporting remains compliant and accurate.
By modernizing banking data infrastructure and processes, banks can improve the reliability and agility of HQLA management. From streamlined data collection and classification to automated reporting and compliance, data modernization supports banks in meeting liquidity requirements in a rapidly changing regulatory landscape.
How Sigmoid’s HQLA solution supports bank liquidity requirements under Basel III
Navigating stringent liquidity regulations under Basel III, including the LCR and FR 2052a, banks need precise asset classification and efficient liquidity risk management. Sigmoid’s HQLA solution offers a powerful framework to meet these needs with transparency and automation, tailored for evolving regulatory demands.
Key features of Sigmoid’s HQLA solution:
- Collateral class and HQLA criteria: Define distinct collateral classes—like government securities and corporate bonds—alongside specific HQLA criteria (e.g., low credit risk, high liquidity, and stability under stress). This structured classification ensures robust liquidity profiles.
- Data models for collateral classes and HQLA: To effectively manage collateral and HQLA classifications, Sigmoid’s solution utilizes structured data models, capturing relevant attributes and establishing clear relationships for each asset type. These models allow banks to automate the classification and management processes, ensuring accurate HQLA reporting across multiple jurisdictions and asset classes.
- Integrated Liquidity Risk Management (LRM): With metrics like the LCR and real-time integration of classification data, the solution supports continuous risk monitoring, enhancing banks’ responsiveness to market fluctuations.
- Automated classification and compliance: Automating the classification engine ensures accuracy across asset types and jurisdictions, while a manual override option allows for flexibility, supporting banks in achieving Basel III compliance with consistency.
By deploying Sigmoid’s HQLA solution, banks can seamlessly cover multi-asset classes, streamline liquidity risk processes, and ensure compliance across regulatory landscapes, all while reinforcing operational efficiency.
Effective liquidity management has become non-negotiable for banks striving to navigate the complexities of the financial landscape. By leveraging real-time insights, automated classification, and comprehensive eligibility criteria, banks can fortify their liquidity positions and proactively mitigate risks. Sigmoid’s HQLA solution not only empowers institutions to meet stringent Basel III requirements but also enhances their operational resilience through advanced data management.
About the author
Shyam Nadig is the Global Head of capital markets at Sigmoid. Shyam has over 25 years of work experience spanning business process operations, service delivery, sales, marketing, and P&L. He is currently running transformational projects around regulatory compliance, cloud modernization, and data quality for key capital markets’ clients. In these initiatives, Shyam leverages Sigmoid’s proprietary methodologies and toolkit to deliver unique value propositions to clients.
Featured blogs
Talk to our experts
Get the best ROI with Sigmoid’s services in data engineering and AI