Building a smarter approach to AI Model evaluation for real business impact
Reading Time: 5 minutes
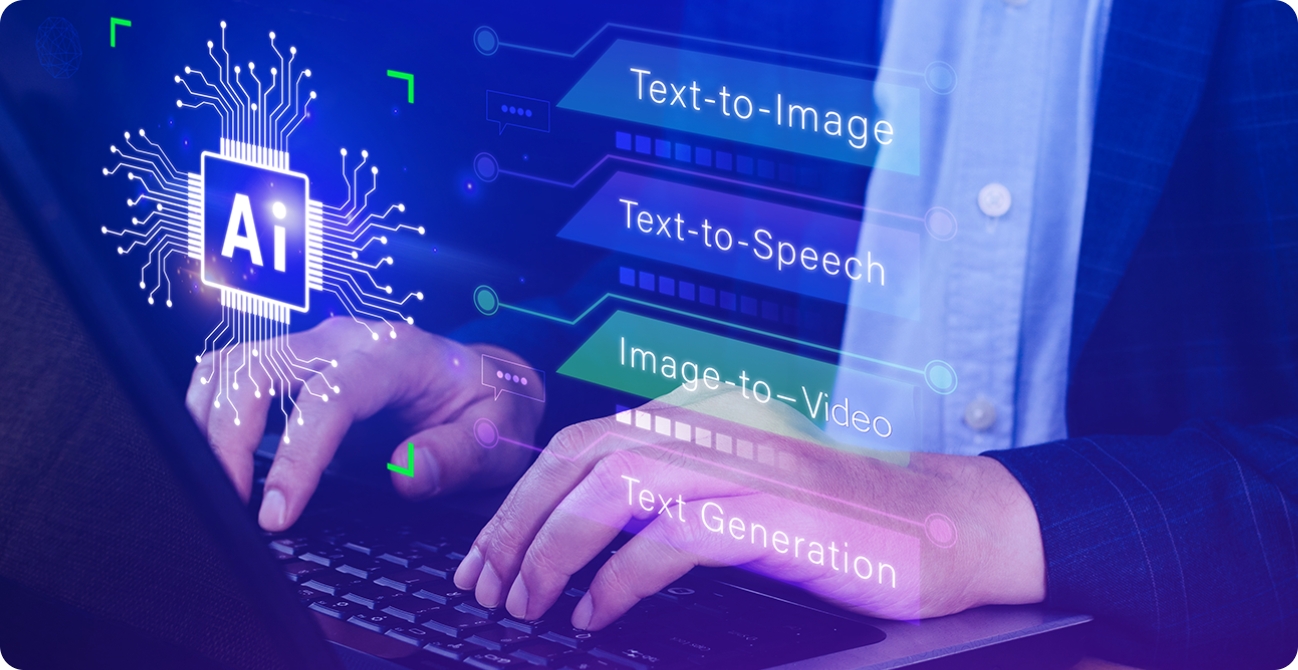
56% of companies have integrated AI into at least one function, underscoring the widespread embrace of AI across industries.¹ However, a study by Boston Consulting Group reveals that 74% of these companies struggle to achieve and scale the desired value from their AI initiatives, highlighting the complexities involved in effective AI adoption.²
The AI landscape is flooded with models boasting different capabilities, costs, and performance benchmarks, making it difficult to identify the most suitable options. Without a structured approach, businesses risk overspending, underutilizing models, or deploying solutions that fail to deliver a meaningful impact.
The complexity of enterprise AI model selection
An overwhelming number of available models often hinders AI adoption in CPG companies, each offering different capabilities, costs, and levels of complexity. Organizations embarking on an AI transformation roadmap often struggle with model selection due to the lack of standardized evaluation criteria.
A successful AI transformation requires balancing innovation with operational feasibility, yet companies face key challenges such as:
- Model overload: The rapid proliferation of AI models makes it difficult to identify the right fit for specific business needs.
- Scalability concerns: AI models must integrate seamlessly into existing infrastructure while remaining adaptable to evolving business demands.
- Cost vs. performance trade-offs: Choosing between high-performing but expensive models and cost-effective alternatives with lower accuracy is a persistent dilemma.
- Compliance & security risks: Ensuring AI models align with data privacy regulations and enterprise security protocols is critical for long-term adoption.
Enterprise architects, data engineering principals, and ML specialists need a structured approach to assess AI models based on performance, scalability, cost-efficiency, and implementation feasibility. Without a clear selection methodology, companies risk investing in models that may not align with their operational needs or long-term AI strategy.
Sigmoid’s approach: A data-driven evaluation framework
To address this challenge, we conducted an in-depth evaluation of 33 AI models, focusing on those widely adopted in enterprise settings and versatile enough to support multiple use cases. All models were sourced from Azure’s repository, ensuring compatibility with enterprise-grade cloud infrastructure, security, and scalability. This structured assessment enables organizations to make informed decisions, balancing technical performance with real-world business impact.
This framework prioritizes business outcomes alongside technical performance, ensuring enterprises choose models that deliver measurable value. Rather than chasing every new model release, Sigmoid advocates for a holistic, metric-driven approach based on the following five key dimensions:
- Performance: Measures the accuracy, coherence, and relevance of model outputs in real-world applications.
- Speed & Resource Use: Evaluates response time, hardware efficiency, and overall computational demand.
- Cost & Value: Considers licensing costs, infrastructure requirements, and overall return on investment.
- Ease of Use: Assesses implementation complexity, developer accessibility, and documentation quality.
- Reliability: Ensures model stability, uptime, and robustness across varied enterprise use cases.
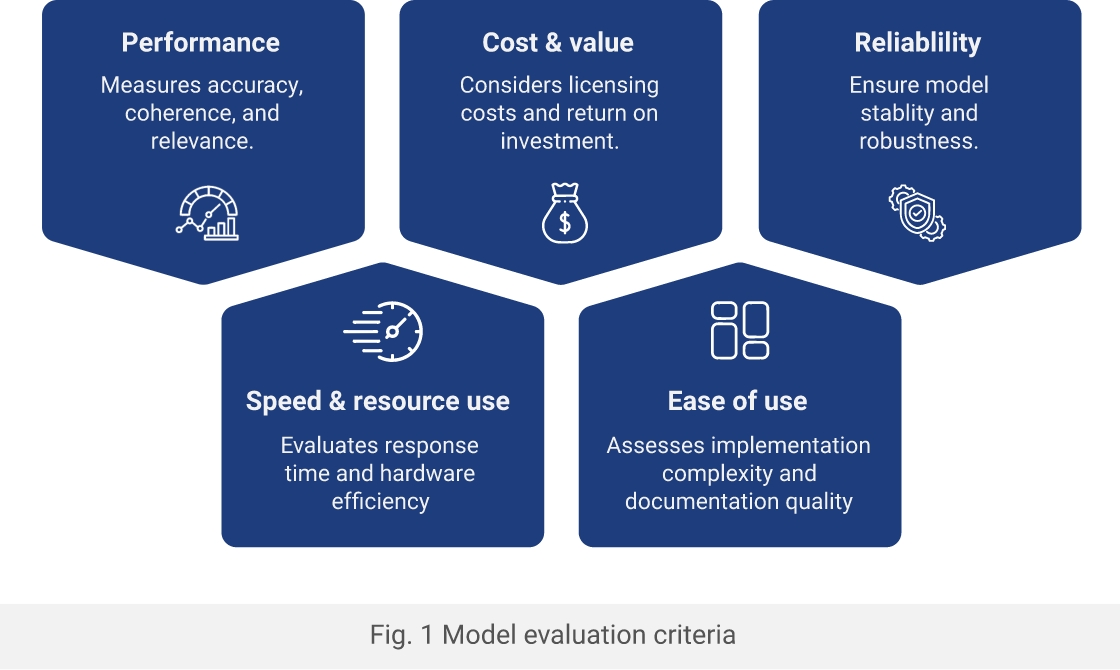
AI model scoring and recommendations
The AI model scoring framework evaluates different models based on the five key parameters mentioned above. Each model is rated on a scale, with a total score reflecting its overall suitability for enterprise applications. The evaluation was conducted through benchmark testing, real-world performance assessments, and cost-benefit analysis, ensuring a balanced view of efficiency, scalability, and practical usability.
After rigorous evaluation, the following models emerged as top contenders for enterprise use:
Model | Performance | Speed & Resource Use | Cost & Value | Ease of Use | Reliability | Total Score |
---|---|---|---|---|---|---|
GPT-3.5 Turbo | 7 | 9 | 8 | 9 | 8 | 8.2 |
GPT-4o | 9 | 7 | 6 | 8 | 9 | 7.8 |
DALL-E 3 | 9 | 6 | 5 | 8 | 8 | 7.2 |
Meta Llama 3.1-405B Instruct | 9 | 5 | 5 | 6 | 8 | 6.6 |
Meta Llama 3.1-405B Base | 9 | 5 | 5 | 5 | 8 | 6.4 |
Key insights from our evaluation
Selecting the most effective AI model isn’t about opting for the biggest or most powerful one—it’s about identifying the right fit for the task at hand. Factors such as cost, efficiency, and domain-specific expertise play a crucial role in determining the ideal choice. Here are the key takeaways from our assessment:
- Bigger isn’t always better: While large models like GPT-4o and Meta Llama 3.1 showcased impressive capabilities, mid-sized models often delivered better value by balancing cost and efficiency for specific tasks.
- Specialized models outperform general-purpose ones: Purpose-built models like Whisper for audio transcription and Codestral for programming excelled in their respective domains, surpassing general AI models.
- Balanced performance drives faster development: Models like GPT-3.5 Turbo, which maintained consistent scores across multiple dimensions, enabled quicker proof-of-concept (POC) and minimum viable product (MVP) development.
- Use case alignment is key: The most effective model varies by business need. For example, text-embedding-3-small is optimized for search applications, while DALL·E 3 remains the best choice for high-quality image generation.
- Skewed scores: Most models in this list scored highly in performance and reliability, which aligns with their leading positions in the industry. This is expected, as the shortlisted vendors are recognized leaders in the AI space.
AI model recommendations by use case
We also mapped each model to specific use cases based on their strengths. This mapping allows the technical teams to quickly identify candidate models to support each business requirement.
Category | Recommended Models |
---|---|
Content Generation (High-Quality) | GPT-4o, GPT-4 Turbo, Mistral Large 2 |
Content Generation (Efficient) | GPT-3.5 Turbo, Mistral 7B Instruct, Meta Llama 3.1-8B Instruct |
Image Generation (Premium) | DALL-E 3 |
Image Generation (Cost-Effective) | DALL-E 2 |
Code and Development (Specialized) | Codestral |
Code and Development (General) | GPT-4o, GPT-4 Turbo |
Search and Embeddings (High Performance) | text-embedding-3-large |
Search and Embeddings (Efficient) | text-embedding-3-small, sentence-transformers/all-MiniLM-L6-v2 |
Voice and Audio | Whisper, GPT-4o Audio |
On-Premise/Privacy-Focused (High Performance) | Meta Llama 3.1-70B Instruct |
On-Premise/Privacy-Focused (Efficient) | Meta Llama 3.1-8B Instruct, Mistral 7B Instruct |
Mobile/Edge Deployment | distilbert-base-uncased, Ministral 8B |
Major takeaways for enterprises
Enterprises must take a strategic approach to AI model selection, considering not just performance but also cost, use-case alignment, and the rapidly evolving AI landscape. Here are the key takeaways for enterprises:
- Context matters: The best AI model depends on the use case. High-performance models may not always be the most cost-effective.
- Balance cost and capability: While GPT-4o and GPT-4 Turbo excel in complex tasks, lighter models like GPT-3.5 Turbo may suffice for many applications.
- Refinement of model choices: Overlapping recommendations indicate that some models can perform multiple tasks well, but careful selection is needed to avoid unnecessary costs.
- AI is evolving: Frequently extending this matrix to include newer models keeps the matrix relevant.
Get the AI Model Evaluation Matrix – Your blueprint for smarter model selection
Note: This evaluation is focused on a selected set of industry-leading AI models to provide a structured comparison. The AI landscape is rapidly evolving, and newer models may offer different capabilities and performance characteristics.
About the authors
Prateek Srivastava, Senior Solutions Architect at Sigmoid, is a seasoned expert with 18 years of experience in Data Strategy Consulting, Big Data Technologies, and Agentic AI. He specializes in designing and implementing AI-driven data ecosystems that power automation, real-time analytics, and highly scalable cloud-native platforms.
Mohammad Shahid Khan, Senior Technical Lead at Sigmoid, is a distinguished expert with 14 years of experience in Big Data Engineering and AI Ops. He has implemented several petabyte-scale solutions across Azure, AWS, and GCP.
Featured blogs
Subscribe to get latest insights
Talk to our experts
Get the best ROI with Sigmoid’s services in data engineering and AI
Featured blogs
Talk to our experts
Get the best ROI with Sigmoid’s services in data engineering and AI