AI enabled real-time analytics improves fraud detection for banks
Reading Time: 5 minutes
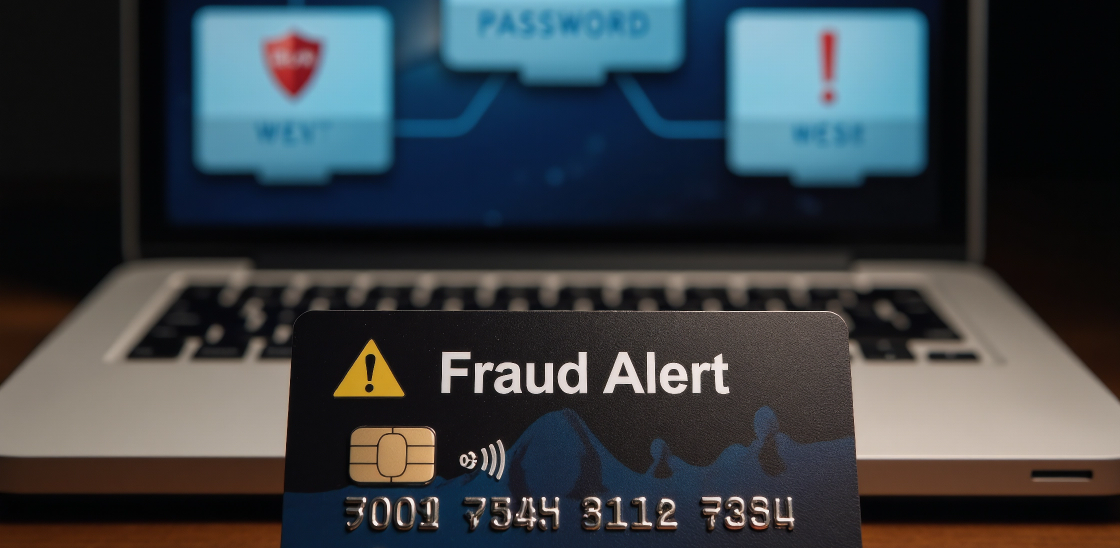
A fraud detection system scanning thousands of transactions can miss subtle red flags hidden in complex patterns. A seemingly legitimate transaction from a long-standing customer in a high-risk location might go unnoticed, only to be flagged days later when the damage is already done.
As fraud tactics evolve, these oversights are becoming a common problem. In 2023, the Federal Trade Commission reported $10 billion in losses from fraud, with $1.2 billion more than the year before.¹ As digital banking, real-time payments, and e-commerce continue to surge, so do the sophistication and speed of fraud schemes. Financial institutions are under increasing pressure to find the right data-driven fraud detection solutions to keep up with evolving threats.
The key to staying ahead? Real-time analytics. By leveraging AI, machine learning, and big data, banks can detect and mitigate fraud in real-time, ensuring that security measures adapt as quickly as fraudsters’ tactics.
Challenges in traditional fraud detection
The rapid growth of online banking or mobile payments has significantly increased the volume, velocity, and variety of financial transactions. With this transformation comes an explosion of sophisticated fraud tactics, which outpace the capabilities of traditional fraud detection systems. Let’s explore the key challenges that highlight these limitations:
- Latency in detection: Traditional systems rely on batch processing, meaning fraud is often detected after the damage has already occurred. With the sheer speed at which fraudulent activities can unfold, delayed detection is no longer acceptable in safeguarding customer assets.
- High false positives: Static, rule-based fraud detection systems rely on pre-defined thresholds and conditions, making them unable to adapt to the complexities of modern fraud patterns. This results in frequent false positives, where legitimate activities are misidentified as fraudulent.
- Scalability issues: The increasing transaction volumes driven by digital transformation strain traditional systems. Legacy infrastructures were not designed to process or analyze data at the scale required for modern fraud detection.
- Lack of contextual insights: Fraud detection requires analyzing transactional, contextual and behavioral information, such as geolocation, device fingerprints, and customer activity patterns. Traditional systems fail to provide a holistic view, leading to missed opportunities for proactive fraud prevention.
Prerequisites to implementing real-time analytics for fraud detection
Real-time analytics enhances fraud detection by delivering significant cost benefits, operational efficiency, and scalability. By detecting threats instantly, it prevents financial losses, reduces false positives, and streamlines fraud investigation processes. Advanced AI-driven models analyze vast datasets in real-time, enabling financial institutions to stay ahead of evolving fraud tactics and strengthen risk management. However, it requires a strategic approach to implementation, ensuring seamless integration, robust model development, and continuous optimization.
- Assess current systems for gaps and improvements
- Selecting the right technology stack
- Developing and refining Machine Learning models
- Seamless integration with core banking systems
- Monitor and optimize for evolving fraud tactics
Before implementing real-time analytics, organizations need to evaluate their existing fraud detection mechanisms. Identifying gaps—such as slow response times, high false positive rates, or limited data integration—helps determine the specific improvements needed.
The effectiveness of real-time fraud detection hinges on the underlying technology. Companies must opt for scalable platforms that support real-time data ingestion, stream processing, and AI-driven analytics. Cloud-based solutions, distributed computing, and event-driven architectures play a crucial role in ensuring smooth operations without latency.
Machine learning models form the core of real-time fraud detection. These models must be trained on diverse and comprehensive datasets, including historical fraud cases, customer behavior, and transaction patterns. Using adaptive learning techniques ensures that models evolve with new fraud tactics, enhancing detection accuracy over time.
For real-time fraud detection to be effective, the system must integrate with core banking infrastructure, payment gateways, and third-party data sources such as geolocation services, device fingerprints, and transaction monitoring platforms, while leveraging cloud transformation for scalability and efficiency.
Fraud tactics continuously evolve, requiring ongoing monitoring and optimization of fraud detection models. Financial institutions should implement real-time dashboards, anomaly detection tools, and automated feedback loops to fine-tune their models based on new fraud patterns.
The role of ML in smarter fraud detection systems
Modern fraud detection in the BFS industry relies on real-time analytics powered by advanced technologies, enabling institutions to detect, analyze, and mitigate fraud as it happens. By leveraging data-driven automation and AI-driven models, financial organizations can stay ahead of increasingly sophisticated fraud tactics. Here’s how real-time analytics is reshaping fraud detection:
- Data ingestion and integration: Real-time fraud detection begins with ingesting vast volumes of transactional data within milliseconds. High-speed, event-driven data streaming ensures that transaction logs, payment activities, and user behavior data are captured instantaneously, providing a strong foundation for real-time analysis. By optimizing data ingestion pipelines, financial institutions can seamlessly integrate structured and unstructured data sources, enabling faster fraud detection.
- AI and Machine Learning Models: Advanced fraud detection systems leverage AI-driven techniques for continuous learning and anomaly detection. Adaptive fraud detection models use:
- Supervised learning for detecting known fraud patterns using labeled datasets.
- Unsupervised learning for anomaly detection in new fraud schemes.
- Reinforcement learning to dynamically adjust fraud detection thresholds based on changing or evolving transaction behaviors.
- Behavioral analytics: Behavior-based fraud detection relies on user profiling and historical transaction monitoring. Suspicious activities, such as an unusually high-value transfer from a rarely used account or an increase in login frequency from a new device, can trigger risk alerts. Risk-scoring mechanisms analyze behavioral deviations in real-time, helping organizations proactively identify potentially fraudulent activities.
- Anomaly detection: Real-time fraud detection leverages advanced analytics and statistical models to pinpoint deviations in transaction patterns. Multi-dimensional analysis allows organizations to detect subtle fraud indicators, ensuring timely intervention before significant losses occur. With intelligent anomaly detection mechanisms, financial institutions can enhance fraud prevention strategies and minimize false positives.
- Real-time alerts and automation: Immediate fraud mitigation is crucial for financial security. Event-driven architectures trigger real-time alerts and automate responses, streamlining fraud case management. Automated rule engines help enforce business logic, ensuring quick decision-making and intervention when fraudulent activities are detected. By integrating automation into fraud detection workflows, financial organizations can improve response times and enhance overall fraud prevention capabilities.
Case Study: Real-time fraud detection
Problem | Solution | Impact |
---|---|---|
The client, one of the largest insurance providers in U.S., struggled with their rule-based fraud detection system, which failed to handle high query volumes, had poor accuracy, and resulted in excessive false positives. The system was inadequate for efficiently flagging fraud and processing valid claims in near real-time. | The solution automated risk assessment by integrating data from 30+ sources, storing high-quality data in Db2, and displaying risk scores via ElasticSearch. A standardized ELT framework improved data quality, enabled fault tolerance, and alerted the compliance team to anomalies for quick action. |
|
Conclusion
As fraud tactics continue to evolve, real-time analytics provides the critical edge needed for financial institutions to stay ahead. By leveraging AI, machine learning, and advanced data integration, BFSI organizations can detect fraud in real-time while reducing false positives, enhancing accuracy, and streamlining fraud prevention processes.
At Sigmoid, we help BFSI organizations implement cutting-edge real-time fraud detection systems that safeguard assets, improve operational efficiency, and protect reputations—ultimately saving money and ensuring long-term trust with customers.
About the author
Bhanu Sashidhar Yerramilli is the Engagement Manager – Consulting, Data Science at Sigmoid. He has over 17 years of experience in Capital Markets and the BFSI sector, bringing deep expertise in financial services, risk management, and strategic decision-making. With a strong foundation in technology and extensive Agile/Scrum experience, he seamlessly integrates financial expertise with agile methodologies, helping companies confidently navigate complex market dynamics.
Featured blogs
Talk to our experts
Get the best ROI with Sigmoid’s services in data engineering and AI