Comparison of ML platforms in an evolving market
Reading Time: 4 minutes
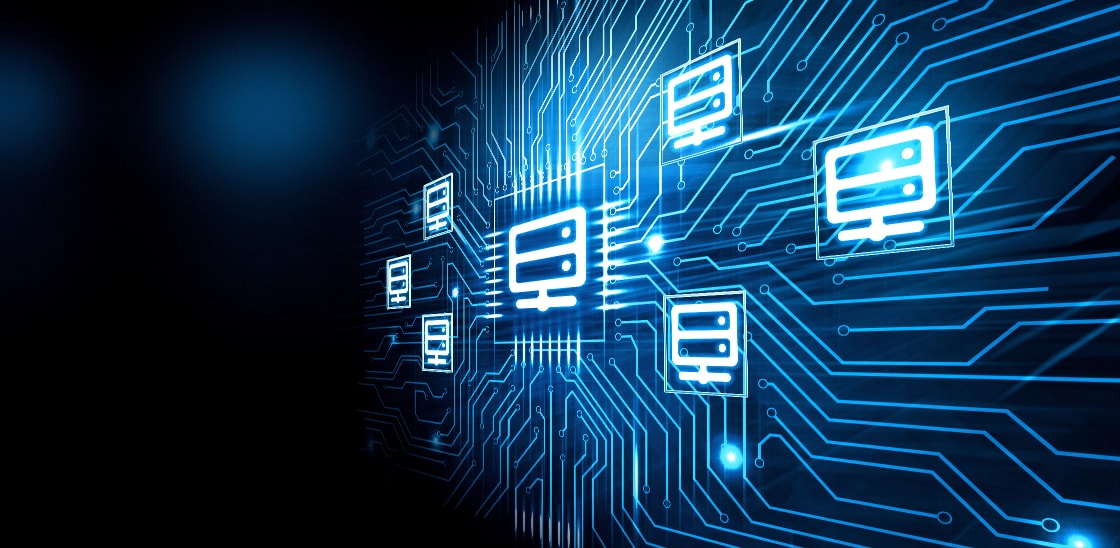
The Evolution of the MLaaS Market
The Machine Learning as a service (MLaaS) market is booming. It is expected to grow to $8.48 billion by 2025, at 43% CAGR. Increased cloud adoption and the need to better comprehend customer behavior are expected to be the driving factors propelling the market, going forward. There’s a clear inclination towards the MLaaS model across industries, given the fact that companies today have an option to select from a wide range of solutions that can cater to diverse business needs. Additionally, the proliferation of IoT, automation and AI-driven systems across industries will only amplify the need for MLaaS as a foundation to all these technologies.
Top Three ML Platforms for Data Excellence
Data Science and cloud machine learning platforms provide companies with the much-needed tools to develop, deploy and monitor ML algorithms. These smart platforms combine data with intelligent, decision-making algorithms that include functionalities for voice recognition, image recognition, natural language processing (NLP) and so on.
There are several ML platforms available in the market. Selecting the right solution, however, can be a daunting task – one that needs thorough research and comparison beyond just the solutions and the core technical capabilities. In order to make the task a little easier, we have profiled the top three cloud ML platforms in one place for review.
IBM Watson
IBM Watson studio or Watson Machine Learning is essentially an amalgamation of IBM cloud services focused on the creation, training, and deployment of neural networks and ML models. By leveraging the Watson Machine Learning platform, companies can develop analytical models and simultaneously train the same with their own data and integrate them into native applications.
Microsoft Azure
Microsoft Azure cloud machine learning platform provides companies with a collaboration platform to develop, test and deploy predictive data solutions. Azure makes the process of creating ML models easy and accessible. This ensures that the developed models can be accessed by a larger audience group within a short time-frame. Additionally, this cloud ML platform also makes it easier for data scientists and engineers to start mining predictive data. This makes it an ideal option for the ones with little to no experience.
Google Cloud AI
Google cloud AI is a fusion of several Google AI/ML based projects like TensorFlow, Kuberflow, and Cloud ML Engine. It includes a complete range of machine learning services like data preparation, data tuning, and data training. Data scientists can leverage this platform to deploy and share ML models and collaborate on its improvement.
A Detailed Comparison of the Three ML Platforms
Features | IBM Watson | Microsoft Azure | Google Cloud AI |
---|---|---|---|
Supported Frameworks | TensorFlow, Spark MLlib, scikit-learn, XGBoost, PyTorch, IBM SPSS, PMML | TensorFlow, scikit-learn, Microsoft Cognitive Toolkit, Spark ML | TensorFlow, scikit-learn, XGBoost, Keras |
Built In Algorithms | Supported. Includes AutoAI and machine learning algorithms that can be deployed for production at scale. | Not Supported. More flexible toward out-of-box algorithms. | Supported with the latest update. However, the implementation is still in beta. |
Speech and text processing APIs | Recognizes 60+ languages and translates in 21 languages | Recognizes 120 languages and translates in 60+ languages | Recognizes 120+ languages and translates in 100+ languages |
UI | Watson UI is aimed primarily toward professional users. Citizen data scientists and business level analysts may find the UX cumbersome. | The Azure GUI is built to visualize each step within the workflow. Less advanced ML teams can explore the UI to understand major methods and models. | An easy to use graphical interface can enable less experienced ML teams to train high-quality models. |
Ease of Adoption | The Watson ML platform can be easily integrated with existing complex analysis and application development systems. However, the platform is definitely more oriented toward professional ML teams. | There is a substantial learning curve in terms to time and effort to be invested while completing a project. This is especially true for less experienced users. | Google ML engine is highly flexible and supports multiple popular algorithms in addition to TensorFlow. Also Data Studio, as one of the most popular data visualization tools caters to data scientists and marketers. |
Cost | Well defined pricing tiers:
|
ML studio with 10gb workspace storage is free for users with a Microsoft account. Standard enterprise-grade workspace can be acquired for US$9.99 per month or US$1 per hour of experimentation. | AI Hub and the notebooks are free. Rest is by subscription (negotiable by contract). Pricing calculator is available on the website but exact numbers can only be figured by contacting Google. |
Rounding Up
Selecting the right cloud ML Platform among the myriad of options available out there can be an overwhelming proposition. Each solution can be differentiated in terms of algorithms, skill sets required to operate the platform and tasks performed. So, how you go about picking that one platform that will cater holistically to all your business needs depends on what your company intends to achieve through the machine learning program. The trick is to rightly align data science with your long term business objectives. Once you achieve this, selecting a platform that ticks all the right boxes will become easier.
About the Author
Vivek Jain is a lead data scientist at Sigmoid with expertise in implementing ML solutions across Hyper personalisation, deep neural networks, demand forecasting, Text analytics, to name a few.
Featured blogs
Subscribe to get latest insights
Talk to our experts
Get the best ROI with Sigmoid’s services in data engineering and AI
Featured blogs
Talk to our experts
Get the best ROI with Sigmoid’s services in data engineering and AI