6 data engineering fundamentals to accelerate generative AI deployment in banking
Reading Time: 4 minutes
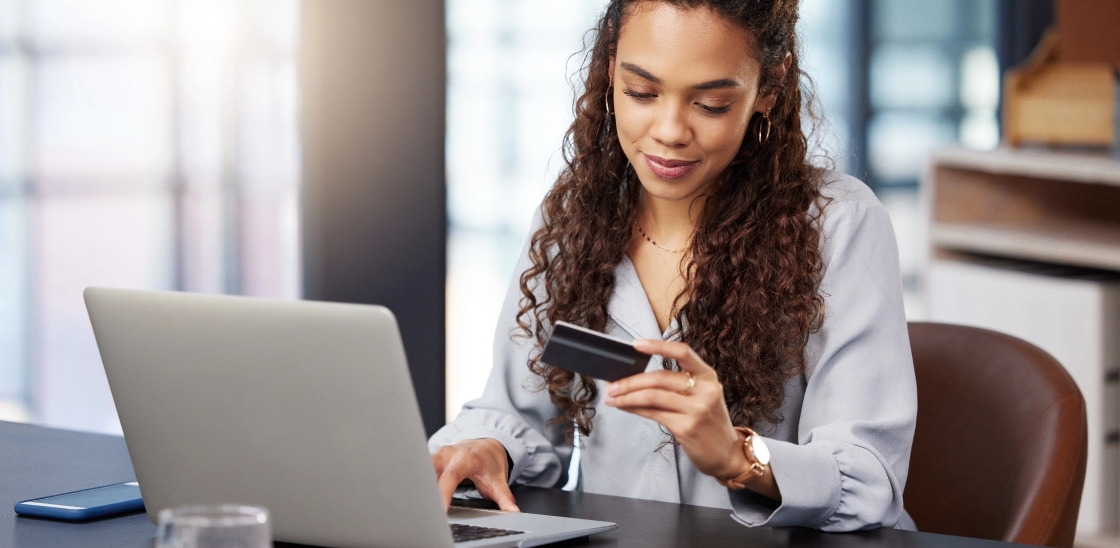
Generative AI-based models are quickly becoming essential solutions for enhancing operational efficiency, improving customer experiences, and mitigating risks in the banking and financial services industry. As banks and financial services embrace the potential of automation and AI-enabled platform operations, it becomes imperative to embed robust data engineering principles that facilitate the seamless deployment and integration of these transformative technologies.
61% of financial institutions say that generative AI will greatly improve efficiency and responsiveness in their operations.1
In this blog, we delve into six essential data engineering best practices tailored to enable the development and deployment of generative AI use cases in the banking industry:

Scalable data infrastructure
Establishing a scalable data infrastructure is essential for accommodating the growing volume of data banks generate. With cloud-based data infrastructures, banks can efficiently handle vast data necessary for training and deploying generative AI models. This infrastructure enables use cases such as proactive fraud detection, personalized customer interactions, and informed risk management.

Continuous Monitoring and LLMOps
To ensure the effectiveness and reliability of generative AI models, banks require robust practices for continuous monitoring and model operations. Continuous monitoring tracks model performance and identifies issues like data quality degradation or model drift. LLMOps (Large Language Model Operations) provide a framework for managing the model’s lifecycle, ensuring smooth deployments, monitoring performance, and maintaining explainability.

API-based approach
API-based architecture enables seamless integration of diverse technologies and systems within the data infrastructure, empowering banks to assemble best-of-breed data solutions. By leveraging APIs, banks can integrate machine learning frameworks, data analytics platforms, and other technologies that facilitate the implementation of generative AI solutions. Additionally, by adopting a “build to reuse” approach banks can design and implement data pipelines that are configurable and adaptable to various business needs within the organization.

Loosely coupled and highly cohesive components
Designing data infrastructure with loosely coupled and highly cohesive components is crucial for ensuring modularity and scalability. Loosely coupled components operate independently, enabling seamless integration of new automation and AI capabilities without disrupting existing systems. On the other hand, highly cohesive components are specialized to perform specific tasks.

Data quality and security
A robust data architecture addressing quality, security, and accessibility is foundational for banks and financial institutions. They must prioritize data quality management to ensure accuracy and consistency while including security measures such as encryption techniques and continuous monitoring. It is also a best practice to follow the FAIR principle for data accessibility.

Data governance framework
For banks deploying generative AI applications, establishing a robust data governance framework is essential to manage the entire lifecycle of data involved. It should clearly define data ownership, implement strict access controls, and maintain comprehensive data lineage to ensure accountability and traceability. Incorporating metadata management tools will enable banks to catalog datasets, model versions, training configurations, and other relevant information, facilitating the reproducibility and auditability of their generative AI solutions.
Conclusion
As generative AI brings new promise for better efficiency and innovation, strategic data engineering will separate leaders from laggards in leveraging its full potential. Banks investing in reusable, API-driven data architectures with robust governance will keep their competitive edge. Generative AI will transform from a buzzword to billions in business value for these forward-thinking institutions.
Featured blogs
Subscribe to get latest insights
Talk to our experts
Get the best ROI with Sigmoid’s services in data engineering and AI
Featured blogs
Talk to our experts
Get the best ROI with Sigmoid’s services in data engineering and AI