3 key components of a data analytics strategy for internal data monetization
Reading Time: 4 minutes
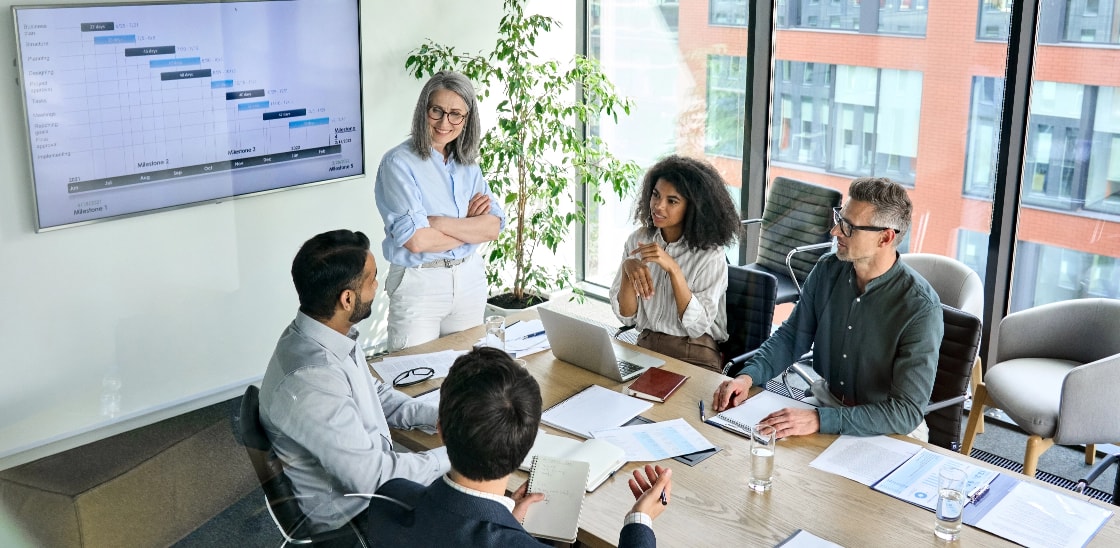
Companies across industries are making data monetization an important part of their strategy to drill down the data and crunch it to create value from it. Gartner predicts that by 2026, over 50% of commercial organizations will have established initial efforts for formal data monetization. If used effectively and exploited timely, the ever-growing enterprise data can reap tremendous profits by increasing revenues or decreasing costs. A study suggests that the global market for data monetization is set to reach $4.1 billion by 2026. An effective data analytics strategy is critical to enable businesses to capitalize on these opportunities.
Various industries such as fintech and retailers are helping companies leverage data collected from payment and customer transactions to create better customer experiences. With greater insights into customers’ behavior, retailers can provide customized insight to marketers and determine what customer segments are most appropriate for other companies, and target ads accordingly.
Identifying opportunities for internal data monetization
For a good data monetization strategy, it is not just enough to own the data until it enables better process optimization and decision-making. Having said that, most organizations struggle to create insights and monetize data despite having established data and analytics practices. The key to overcoming these challenges lies in implementing an integrated data analytics strategy across the organizations.
One of the biggest challenges with data is that it is fragmented and exists in silos. Without a unified data analytics strategy, different groups in an organization work in a fragmented manner with their own data and goals, often lacking a common objective. This makes it difficult to use the data to gain any real benefits.
3 steps to enable data monetization
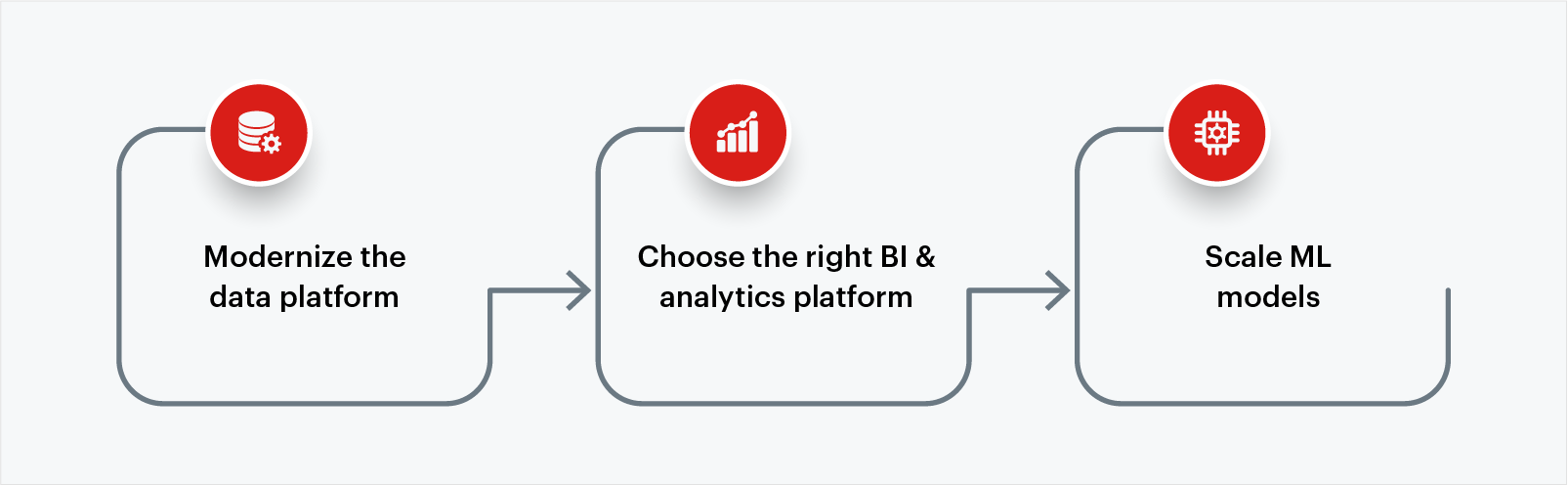
Modernize the data platform
To take advantage of data monetization internally, start by reviewing your data strategy, defining a common goal, and uncovering areas for improvement and optimization. Bringing data existing in different parts of the organization together is another challenge. For instance, CPGs have access to rich data streams across social media, marketing platforms, CRMS, demographic data, and more but it largely remains unexplored. Examining the available data streams and making them available quickly, easily, and efficiently is the next important step.
Strong data engineering practices improve data quality and discover new smarter information that matters to the organization by cleaning and de-duplicating data, reducing the average query processing time, and generating faster insights. It provides the right expertise to build and streamline data processing pipelines, and modernize the data platform to enable rapid AI adoption. Working with data experts who can manage and engineer data for better accessibility is crucial for leveraging it for monetization as it can make data processing powerful and reliable.
Choose the right BI and analytics platform
A data monetization strategy aims at extracting insights from big data sets such as customer sentiment, sales data, product sales, etc. The insights generated from analytical methods can help companies determine what products are consumers buying and how they could sell more products. Granular insights like these can help companies increase marketing efficiency, for instance, and enable them to attribute every single spend to revenue increasing the overall sales.
Data-based insights can help companies deliver meaningful services, assess customer demands, and optimize production in an informed manner. A centralized database such as the data lake solution along with data visualization and BI tools facilitates storage and analysis of data to generate insights.
Scale ML models
The lack of advanced data and AI successes for various enterprises may stem primarily from scaling challenges. Even when companies have pre-processed data its translation into full business impact can be challenging. To realize business gains, just deploying machine learning models into workflows is not enough, it needs to be operationalized and scaled with the right mix of MLOps, data science, data engineering, and DataOps practices. Many organizations find the modeling part easy but spend a lot of time running and scaling them, ending up spending a lot of time and financial resources on duplicating models.
Managing the right compute, distributor framework, model drift analysis and hyperparameter tuning at that scale remains a major challenge. By implementing a multi-step approach to machine learning such as building a logical testing framework, automating model drift testing, and feature engineering the pipelines can scale up the models. Enabling AI engineering can improve maintenance cost, speed, and performance while horizontally scaling the system. With the model stability and improved operations, companies can benefit in the long run with improved model output and saving resources on model building.
Conclusion
To be able to find the connection between data and revenue is one of the key benefits of internal data monetization. With a strong data foundation, data infrastructure, and productionization of machine learning models at scale, companies can leverage quality data to perform competitive cost benefits and increase revenue.
While setting out a path to data monetization can be tough, companies can leverage data specialists to build data platforms and engineering capabilities to process data from across sources. External specialists can bring experience in architecting, configuring, and monitoring complex data environments and use advanced tools to design high-throughput database environments that facilitate distributed data access. Building scalable infrastructure that is secured and enabled integrations across various tools and platforms can create a perfect scenario for companies to set up a monetization strategy.
Featured blogs
Subscribe to get latest insights
Talk to our experts
Get the best ROI with Sigmoid’s services in data engineering and AI
Featured blogs
Talk to our experts
Get the best ROI with Sigmoid’s services in data engineering and AI